Description
Irrigation represents one of the most impacting human intervention in the water cycle considering that irrigated agriculture accounts for about 70 percent of water withdrawn worldwide from lakes, rivers and aquifers. Indeed, the expected increasing population in the future and consequently the increasing demand of food will require an improvement in the management of irrigation with a strong interest in reducing water withdrawals and increasing irrigation efficiency. In this context, the knowledge of the distribution and extent of irrigated areas as well as the amount of water used for irrigation plays a central role for both modelling irrigation water requirements at global scale and quantifying the impact of irrigation on climate, river discharge and groundwater depletion. On the other hand, obtaining high-quality information about the actual irrigated areas worldwide is challenging especially in terms of quantification of the water actually used for irrigation. This problem is well known in most of large scale hydrological/land surface models that do not include or are characterized by simplified irrigation schemes. The main goal of Irrigation+ project is to explore, develop and validate advanced Earth Observation-based algorithms and techniques for irrigation mapping, quantification and detection of seasonal timing of irrigation from field to regional/global scale. This especially capitalizing on the latest advances in Earth Observations and on the advent of the European Space Agency (ESA) Sentinel missions in synergy with other data types and models. The production of local to global satellite databases at different scales will be the basis to operationalization of irrigation products followed by impact assessment studies.
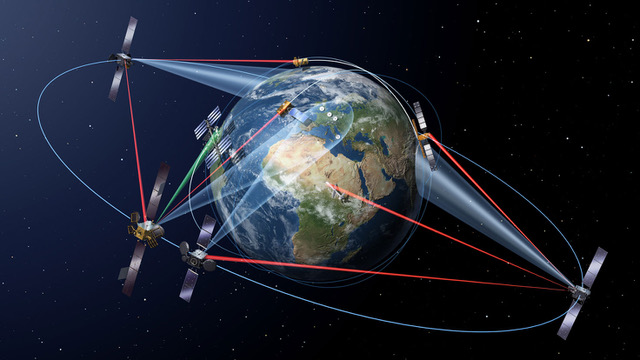
Period
March 2020 – October 2022
News
- How much water is used for irrigation?
Feeding a growing global population is a major concern, but efforts to grow more food will place an added burden on precious supplies of freshwater. We have developed a new approach for using measurements of soil moisture from space to understand how much water is used for agriculture and potentially help farmers manage consumption.
We now plan to extend the study to explore the potential offered by the high-resolution data from the new Copernicus mission, Sentinel-1, jointly with the very high-resolution optical observations from Sentinel-2.Including Sentinel missions in our study may change the spatial and temporal scales at which we can observe irrigation from space. I am guessing that with more research these new high-resolution products will be a game changer in the near future to advance our capacity to better monitor irrigation over agricultural lands.
We have already published the first paper outlining our approach:
“How much water is used for irrigation? A new approach exploiting coarse resolution satellite soil moisture products” International Journal of Applied Earth Observation and Geoinformation
by L. Brocca, A. Tarpanelli, P. Filippucci W. Dorigo F. Zaussinger, A. Gruber and D. Fernández-PrietoWe have also raised the interest of MEDIA in the ESA website, the Science Trends and we give an interview (in Italian) for “Economia Cristiana“.
We have won the “BayWa Smart Farming Challenge” at 2018 COPERNICUS MASTER for the idea “SPACE-IRR – How Much Water for Irrigation?”
Partners
The consortium is leaded by CNR-IRPI (Perugia, Italy) with the collaboration of 6 partners:
- eLEAF company (Netherlands)
- KU Leuven (Belgium)
- Vienna University of Technology (TU Wien, Austria)
- SPIRE GLOBAL LUXEMBOURG S.A.R.L. (Luxembourg)
- Universitat Ramon Llull, Observatoir de l’Ebre (Spain)
- Université Toulouse III Paul Sabatier (France)
Scientific Papers (Project publications)
- Zappa, L., Schlaffer, S., Brocca, L., Vreugdenhil, M., Nendel, C., Dorigo, W. (2022). How accurately can we retrieve irrigation timing and water amounts from (satellite) soil moisture? International Journal of Applied Earth Observation and Geoinformation, 113, 102979, doi:10.1016/j.jag.2022.102979.
- Modanesi, M., Massari, M., Bechtold, M., Lievens, H., Tarpanelli, A., Brocca, L., Zappa, L., De Lannoy,G.J.M. (2022). Challenges and benefits of quantifying irrigation through the assimilation of Sentinel-1 backscatter observations into Noah-MP. Hydrology and Earth System Sciences Discussions, preprint, in press, doi:10.5194/hess-2022-61.
- Brombacher, J., Rezende de Oliveira Silva, I., Degen, J., Pelgrum H. (2022). A novel evapotranspiration based irrigation quantification method using the hydrological similar pixels algorithm. Agricultural Water Management, 267, 107602, doi:10.1016/j.agwat.2022.107602.
- Elwan, E., Le page, M., Jarlan, L., Baghdadi, N., Brocca, L., Modanesi, S., Dari, J., Quintana Segui, P., Zribi, M. (2022). Irrigation mapping on two contrasted climatic contexts using Sentinel-1 and Sentinel-2 data. Water, 14(5), 804, doi:10.3390/w14050804.
- Dari, J., Brocca, L., Quintana-Seguí, P., Casadei, S., Escorihuela, M.J., Stefan, V., Morbidelli, R. (2022). Double-scale analysis on the detectability of irrigation signals from remote sensing soil moisture over an area with complex topography in central Italy. Advances in Water Resources, 161, 104130, https://doi.org/10.1016/j.advwatres.2022.104130
- Dari, J., Quintana-Seguí, P., Morbidelli, R., Saltalippi, C., Flammini, A., Giugliarelli, E., Escorihuela, M.J., Stefan, V., Brocca, L. (2022). Irrigation estimates from space: implementation of different approaches to model the evapotranspiration contribution within a soil-moisture-based inversion algorithm. Agricultural Water Management, 265, 107537, https://doi.org/10.1016/j.agwat.2022.107537
- Ayari E, Kassouk Z, Lili-Chabaane Z, Baghdadi N, Zribi M. (2022) Investigation of Multi-Frequency SAR Data to Retrieve the Soil Moisture within a Drip Irrigation Context Using Modified Water Cloud Model. Sensors, 22(2), 580, https://doi.org/10.3390/s22020580
- Massari, C., Modanesi, S., Dari, J., Gruber, A., De Lannoy, G.J.M., Girotto, M., Quintana-Seguí, P., Le Page, M., Jarlan, L., Zribi, M., Ouaadi, N., Vreugdenhil, M., Zappa, L., Dorigo, W., Wagner, W., Brombacher, J., Pelgrum, H., Jaquot, P., Freeman, V., Volden, E., Fernandez Prieto, D., Tarpanelli, A., Barbetta, S., Brocca, L. (2021). A review of irrigation information retrievals from space and their utility for users. Remote Sensing, 13(20), 4112, https://doi.org/10.3390/rs13204112
- Modanesi, S., Massari, C., Gruber, A., Lievens, H., Tarpanelli, A., Morbidelli, R., and De Lannoy, G. J. M. (2021). Optimizing a backscatter forward operator using Sentinel-1 data over irrigated land, Hydrology and Earth System Sciences, 25, 6283-6307, https://doi.org/10.5194/hess-25-6283-2021
- Dorigo, W., … Brocca, L., … Wagner, W., Yu, L., Zappa, L., …& Aich, V. (2021). Closing the water cycle from observations across scales: Where do we stand?, Bulletin of the American Meteorological Society, 102(10), E1897–E1935, https://doi.org/10.1175/BAMS-D-19-0316.1
- Ouaadi, Nadia, Lionel Jarlan, Saïd Khabba, Jamal Ezzahar, Michel Le Page, and Olivier Merlin (2021). Irrigation Amounts and Timing Retrieval through Data Assimilation of Surface Soil Moisture into the FAO-56 Approach in the South Mediterranean Region. Remote Sensing, 13(14), 2667, https://doi.org/10.3390/rs13142667
- Dari, J., Quintana-Seguí, P., Escorihuela, M.J., Stefan, V., Brocca, L., Morbidelli, R. (2021). Detecting and mapping irrigated areas in a Mediterranean environment by using remote sensing soil moisture and a land surface model. Journal of Hydrology, 56, 126129, https://doi.org/10.1016/j.jhydrol.2021.126129
- Zappa, L., Schlaffer, S., Bauer-Marschallinger, B., Nendel, C., Zimmerman, B., & Dorigo, W. (2021). Detection and Quantification of Irrigation Water Amounts at 500 m Using Sentinel-1 Surface Soil Moisture. Remote Sensing, 13(9), 1727, https://doi.org/10.3390/rs13091727
- Zribi, M., Foucras, M., Baghdadi, N., Demarty, J., Muddu, S. (2020). A new reflectivity index for the retrieval of surface soil moisture from radar data. IEEE Journal of Selected Topics in Applied Earth Observations and Remote Sensing, 14, 818-826, https://doi.org/10.1109/JSTARS.2020.3033132
- Le Page, M., Jarlan, L., El Hajj, M. M., Zribi, M., Baghdadi, N., Boone, A. (2020). Potential for the Detection of Irrigation Events on Maize Plots Using Sentinel-1 Soil Moisture Products. Remote Sensing, 12(10), 1621, https://doi.org/10.3390/rs12101621
- Dari, J., Brocca, L., Quintana-Seguí, P., Escorihuela, M.J., Stefan, V., Morbidelli, R. (2020). Exploiting high-resolution remote sensing soil moisture to estimate irrigation water amounts over a Mediterranean region. Remote Sensing, 12(16), 2593, https://doi.org/10.3390/rs12162593
Reference Papers
- Brocca, L., Tarpanelli, A., Filippucci, P., Dorigo, W., Zaussinger, F., Gruber, A., Fernández-Prieto, D. (2018). How much water is used for irrigation? A new approach exploiting coarse resolution satellite soil moisture products. International Journal of Applied Earth Observation and Geoinformation, 73C, 752-766, https://doi.org/10.1016/j.jag.2018.08.023
- Filippucci, P., Tarpanelli, A., Massari, C., Serafini, A., Strati, V., Alberi, M., Raptis, K.G.C., Mantovani, F., Brocca, L. (2020). Soil moisture as a potential variable for tracking and quantifying irrigation: a case study with proximal gamma-ray spectroscopy data. Advances in Water Resources, 136, 103502, https://doi.org/10.1016/j.advwatres.2019.103502
- Modanesi, S., Massari, C., Camici, S., Brocca, L., Amarnath, G. (2020). Do satellite surface soil moisture observations better retain information about crop-yield variability in drought conditions? Water Resources Research, 56(2), e2019WR025855, https://doi.org/10.1029/2019WR025855
- Jalilvand, E., Tajrishy, M., Hashemi, S.A.G., Brocca, L. (2019). Quantification of irrigation water using remote sensing of soil moisture in a semi-arid region. Remote Sensing of Environment, 231, 111226, https://doi.org/10.1016/j.rse.2019.111226
- Zaussinger, F., Dorigo, W., Gruber, A., Tarpanelli, A., Filippucci, P., Brocca, L. (2019). Estimating irrigation water use over the contiguous United States by combining satellite and reanalysis soil moisture data. Hydrology and Earth System Sciences, 23, 897-923, https://doi.org/10.5194/hess-23-897-2019
- Brocca, L., Ciabatta, L., Massari, C., Camici, S., Tarpanelli, A. (2017). Soil moisture for hydrological applications: open questions and new opportunities. Water, 9(2), 140, https://doi.org/10.3390/w9020140
Results
HSP method developed by e-LEAF and based on an update of SEBAL algorithm by Bastiaanssen et al. (2012), is used within the Irrigation+ project to calculate Incremental ET due to irrigation. This allows to calculate water use per field as shown below for the Hex Valley in South Africa.
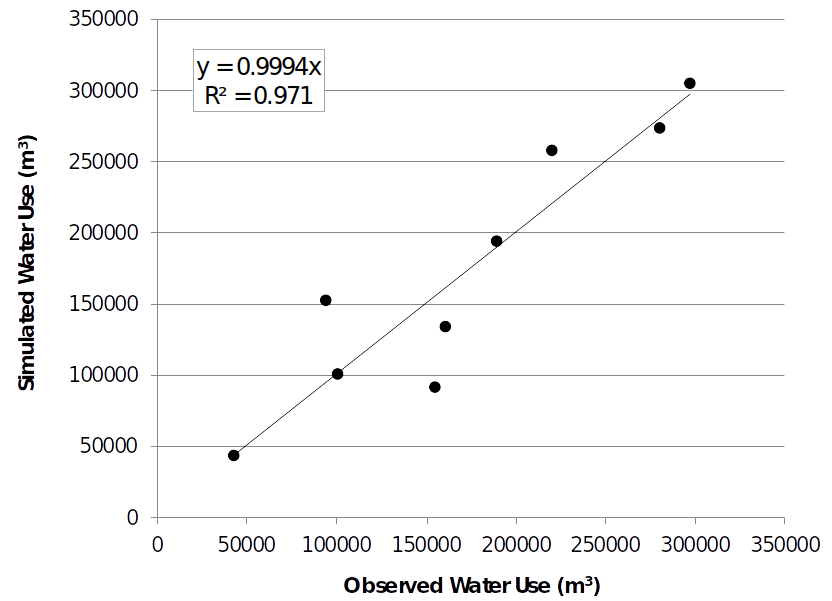
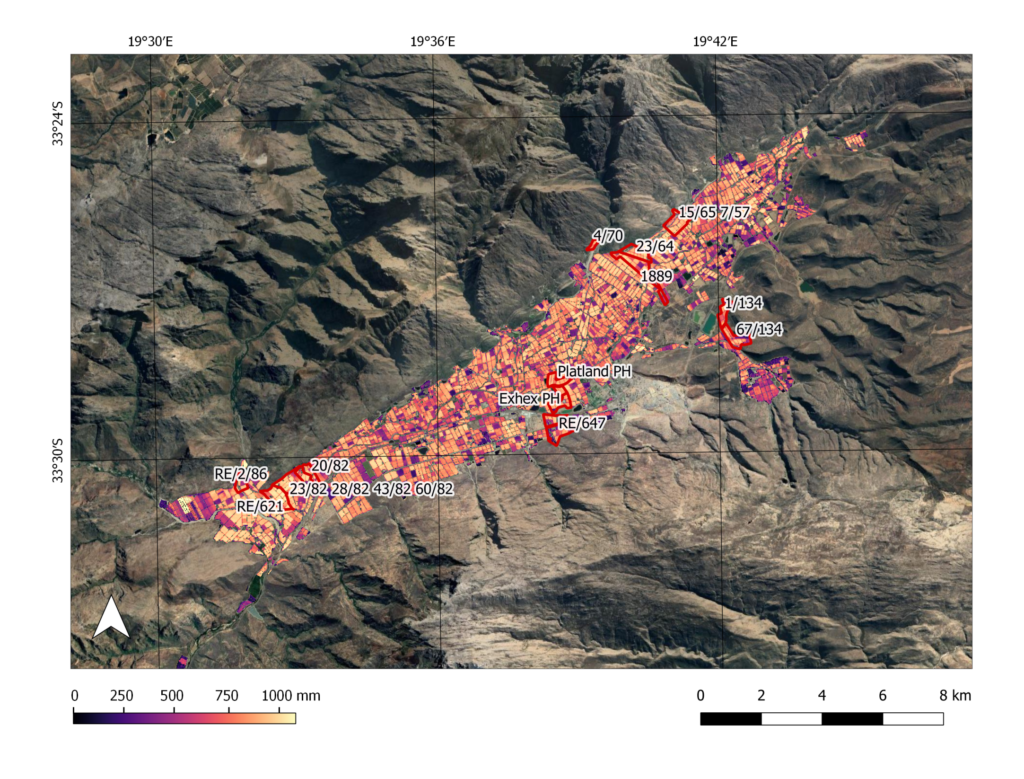
Irrigation amounts at 1 km spatial resolution over North-East of Spain retrieved by downscaled ESA SMOS (Soil Moisture and Ocean Salinity) soil moisture and referring to the period 2011-2015. The irrigation estimates have been obtained through the SM-based inversion algorithm. The spatial scale at which irrigation occurs over the pilot agricultural areas and the fragmentation of the irrigated fields introduce not-negligible uncertainties in the analysis at 1 km spatial resolution that make the adoption of higher resolution data necessary.
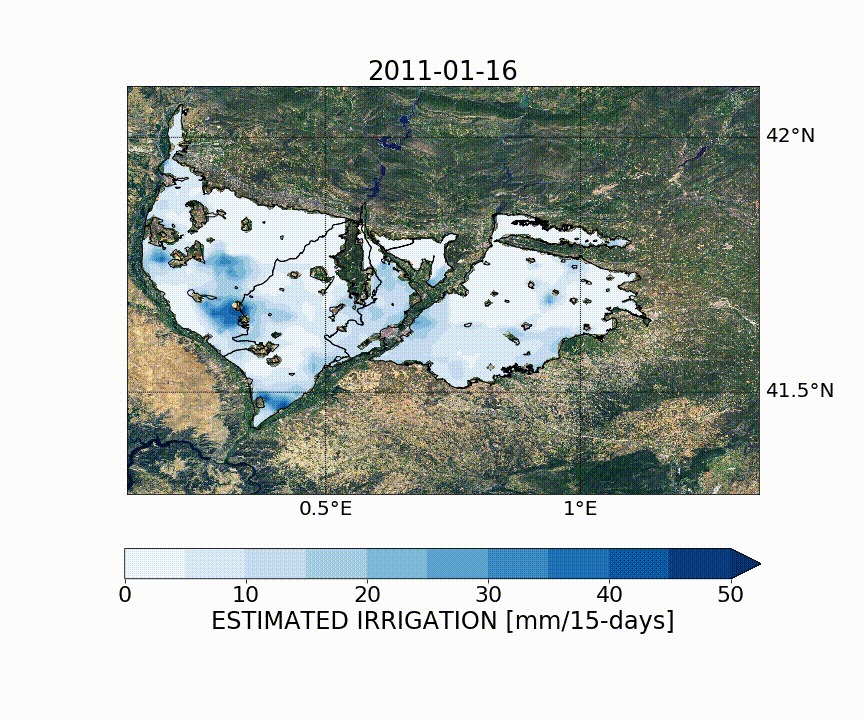
Preliminary Data assimilation experiment in which the Sentinel-1 σ0 VV is used to update the SM state variable into the Noah-MP LSM running with a sprinkler irrigation scheme activated. The figure shows comparisons between the open loop (OL) and DA runs in terms of SM (upper plot), LAI (central plot) and irrigation (bottom plot). The assimilation of the Sentinel-1 σ0 VV modify the SM and LAI dynamics. In terms of irrigation the DA experiment improves the performance as compared to the OL run, provading a reduction in the total amount of irrigated water, closer to the observed in situ irrigation.
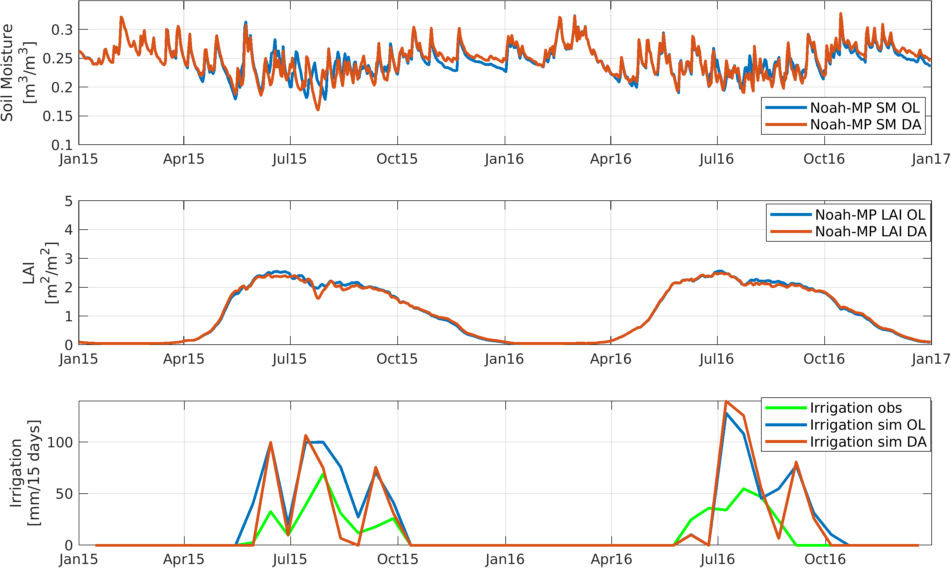
Datasets and Deliverables
Datasets
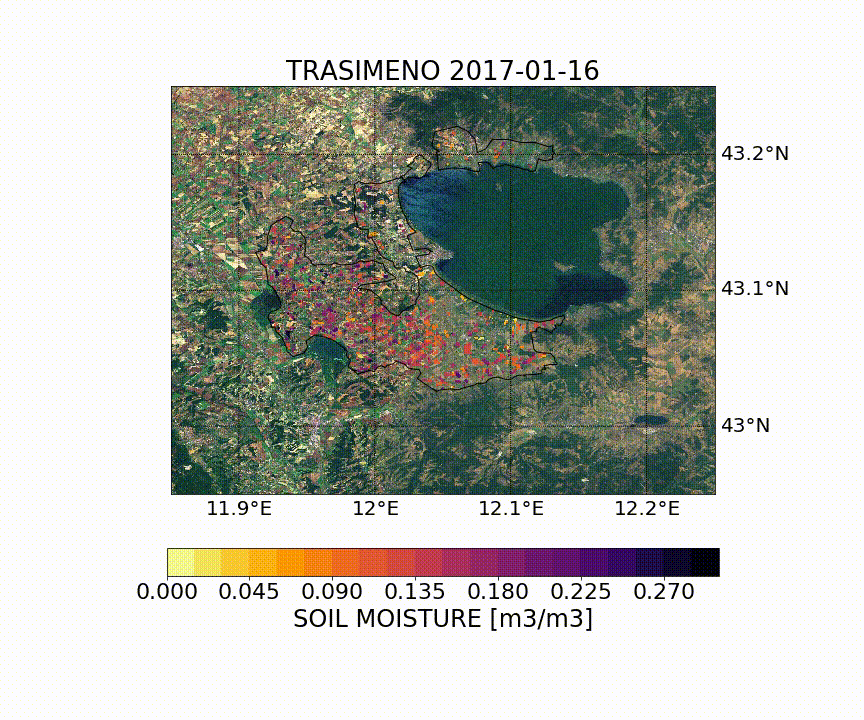
The soil moisture product Theia developed by Toulouse is a very high spatial resolution product for which data are provided at plot scale. The soil moisture inversion algorithm is represented by the Water Cloud Model (Attema & Ulaby, 1978) plus the physical backscattering model IEM (Integral Equation Model), specifically used to compute the σ0soil contribution. The Sentinel-1 backscatter (σ0) VV polarization and the NDVI (Normalized Differential Vegetation Index) obtained from Sentinel-2 images are used as input in the algorithm. Additional input is represented by the land cover maps used to mask the agricultural areas (and the grasslands).
Deliverables
Requirement Baseline Report D1.1
Task 3 Mid-Term Technical Note Report D3.1
Contacts
Luca Brocca (luca.brocca@irpi.cnr.it)
Angelica Tarpanelli (angelica.tarpanelli@irpi.cnr.it)
Sara Modanesi (sara.modanesi@irpi.cnr.it)
Christian Massari (christian.massari@irpi.cnr.it)
Jacopo Dari (jacopo.dari@unipg.it)